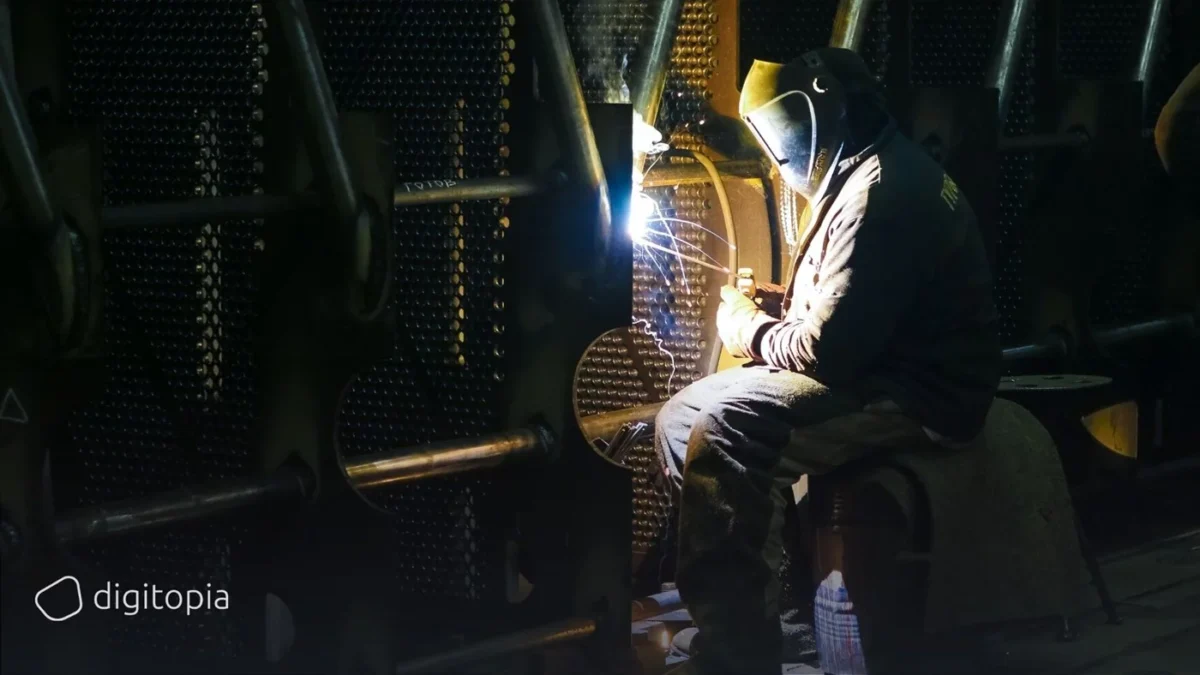
What happens when a machine that is at the heart of your operations fails unexpectedly? How much of an inconvenience is it? How do you fix it? How long does it take?
Defects and common wear and tear are easier to detect on some parts of big complex machines than others. A dent on the body of a machine and a crack in a layer of a bearing in an engine is not equally visible.
The consequences of problems resulting from issues with these parts also differ. For instance, a dent on the surface of a machine might not be detrimental to production efficiency or operational continuity. On the other hand, it can create a butterfly effect when a bearing breaks.
Imagine a bearing in an engine of a machine starts to crack. It could be due to the duration of use, amount of pressure, friction, heat, or anything you can name. You don’t notice the crack immediately because the bearing continues to work, albeit with gradually decreasing efficiency. In the best case, you notice the drop in the machine’s performance after a while and try to find the problem. In the worst case, since it happens so gradually, you don’t notice the change in efficiency and only realize that there is a problem when the bearing breaks.
In either case, you don’t know the source of the problem. The more integrated the part is, the longer it takes to find. You need to stop the machine, or your operations altogether, to find the issue. Since the interruption is unplanned, you enter emergency mode, scramble to find technical experts to fix it and order spare parts. In the meanwhile, your operations continue to be affected, also affecting your production schedules, shipment calendars, and customer satisfaction. Depending on your relationship with your customers, your place in the industry, and your emergency readiness, it could be possible to manage the situation with a very strong relationship management approach or you could lose customers if the issue takes time to be resolved.
You should not settle for either of these cases. Operational excellence and customer focus should not be compromised. For this, you need to be proactive and ready for emergencies. Predictive maintenance offers a third, more proactive alternative.
You Accrue More Than Just Maintenance Costs When a Critical Machine Fails
When a critical machine fails, it affects the overall downtime, operational schedules are interrupted, and you lose out on potential production output. You lose time while trying to figure out the source of the failure, waiting for the experts to investigate the asset, and waiting for the arrival of spare parts to replace the dysfunctional parts. There may be added waste due to a defect in a batch of the production caused by the failure or extra strain on other machines while trying to make up for the production loss. Both the unexpected nature of the failure and exclusive dependence on human capabilities to monitor and fix the machines make you more vulnerable against risks.
For manufacturing enterprises, it is crucial to have a strategy for emergency response and maintenance. Besides a clearly defined strategy, investing in digital capabilities and effectively utilizing your digital superpowers will reduce the risks and keep you better prepared in the event of failures.
Don’t Know Where to Start? Take One Step at a Time: Define Your Maintenance Strategy
There are several factors to consider while defining your maintenance strategy. Understanding different parts and stages of your operations and how they relate to each other is the first step. This allows you to assess risks more accurately and prioritize prevention measures accordingly.
In assessing risks, consider the probability of failure and the extent of the impact that the failure generates: What would be the impact of the breakdown on different units? On the overall operations? What would be the magnitude of repair or difficulty of maintenance?
Different degrees of maintenance correspond to different needs:
- Reactive maintenance entails responding to a malfunction or breakage after it happens. Such run-to-fail approach works best for maintaining assets that are easy to fix and parts that can be stored without much cost.
- Preventive maintenance is more proactive than reactive maintenance as it takes place before things break. Preventive maintenance can be tied to a time or use-based schedule where the assets are maintained at fixed intervals, such as after a certain period or after certain number of cycles. Preventive maintenance helps to avoid costs incurred by unexpected failures. On the other hand, it is not a very efficient approach as some perfectly functioning parts might be changed during the process.
- Condition-based maintenance is a more advanced version of preventive maintenance as it is based on an asset’s condition (i.e., when there is an observable decline in an assets efficiency or there is wear and tear). Here, data and signals are constantly monitored. Intervention happens when the values diverge from the pre-determined range that indicates the acceptable values for operation. Condition-based maintenance is much more efficient than schedule-based preventive maintenance as it uses data to track an asset’s performance and allows users to maximize part lifetimes.
- Predictive maintenance is done through software where data is analyzed to predict future failures. Predictive maintenance is different from condition-based maintenance. Condition-based maintenance detects anomalies and flags situations/assets when the readings fall outside of pre-defined parameters. On the other hand, given the trends in data, predictive maintenance uses artificial intelligence and machine learning (ML) algorithms to predict the likelihood of malfunction in the future and raises a flag before the relevant metrics start falling outside of the pre-defined parameters.
A broken light bulb does not impact business continuity the same way a broken machine in the production line does. It also consumes less time and resources to change a light bulb than to replace a unique part of a complex machine. Thus, you would not approach the two instances with the same maintenance strategy. While reactive maintenance is the best response to fixing a broken light bulb, a predictive approach should be the mode of operation that you strive for when maintaining more complex assets.
Predictive Maintenance Will Improve Your Operations, Ensure Continuity and Maximum Efficiency; But First, You Need to Prepare the Infrastructure to Reap the Benefits
Its multitude of benefits make predictive maintenance desirable. First, since it predicts future failure and gives early warnings based on the performance of a currently functional asset, it reduces downtime. Furthermore, although setting up predictive maintenance systems requires some CAPEX, predictive maintenance lowers maintenance costs in the long run. The longer you run the AI and ML algorithms, the more they learn and the more accurate they become. This provides you with much more precise diagnoses and allows you to optimize the efficiency of your assets. Also, predictive maintenance indirectly helps you save on costs incurred by operational interruption due to malfunction.
Like many cutting-edge approaches, employing predictive maintenance carries some challenges. The first challenge is the lack of a maintenance strategy. Every enterprise emphasizes productivity and continuity of operations as greatly important. Issues of such importance require deliberate strategies and tangible steps. The more enterprises close the gap between intention and action, the better their operations benefit from the leadership’s vision. Another important challenge is related to patience and persistence. Predictive maintenance uses technology that depends on data. Collecting big volumes of accurate data to train the AI and ML algorithms requires patience. Looking forward and ensuring your continuous investment in predictive maintenance could work wonders and lead the benefits to outweigh costs sooner than you expect.
From Technology to Strategic Collaborations: You Need to be Active on Multiple Fronts in Utilizing Predictive Maintenance
Employing predictive maintenance is both an operational issue and a business decision as it requires upfront investment. To begin with, you need the necessary hardware, such as sensors, as well as the infrastructure and tools for connectivity, storage, and communication security. Data is the backbone of predictive maintenance. You need to be able to collect and store data in large volumes. The data feed also needs to be high quality, well tagged, and rich. You need to have the computing power and tools that uses AI and ML to process the collected data.
There is a variety of predictive maintenance software that offer user-friendly and easy-to-navigate interfaces, and ready-to-go analyses. In order for predictive maintenance to work, there needs to be a period of learning and experimentation to train the AI models. The more data points collected, the more accurate and precise the estimations will be. This requires time and close monitoring. Here, your experienced operators are the best supervisors since they know the machinery, system, and operations the best. Their insights and input are very valuable in training the AI to look for nuances and specific parameters, and eventually to improve on them. Once you are satisfied with the experiment results, then the AI and ML operations ensure the continuity of the process.
Interaction and collaboration with your ecosystem are crucial to get the optimal benefit from predictive maintenance. Collaboration also helps to overcome the challenges presented by the lack of data. Collaboration can happen on multiple levels and with multiple stakeholders. The manufacturer of your most complex equipment is a great source of data on its working and performance. Data from them can make great headway for the predictive maintenance software. Your service partners can have access to various enterprises and information, and even your competitors who use similar assets can provide data on the efficiency of their equipment. Providers of AI and predictive maintenance solutions can serve as a hub, connecting need, information to address the need, and relevant solution.
It is important to highlight that the above-mentioned collaboration does not mean sharing sensitive business information. On the contrary, when done right, sharing anonymized information, and contributing to the efforts to optimize services and algorithms benefits everyone, including manufacturers and operators of the assets, as well as maintenance providers.
Are You Ready to Take the Next Step?
Understanding your operations and knowing your maintenance options saves you time, allows you to plan ahead, and be more resilient overall. Here, patience and persistence are the most important ingredients you need to reap the benefits.
In our Digital Maturity Index (DMI) we measure an enterprise’s maturity with respect to 6 dimensions on 5 levels. One pillar of operational maturity is maintenance activities. An enterprise at Level 5 uses a combination of maintenance strategies, has invested in predictive maintenance tools, runs maintenance operations smoothly, and almost never faces surprises that threaten the continuity of operations.
At Digitopia, we help enterprises to make the decisions to get to this point. Our DMI provides a holistic picture of an organization’s digital state along with industry benchmarks. We measure, we transform, and we create impact. Let’s talk.