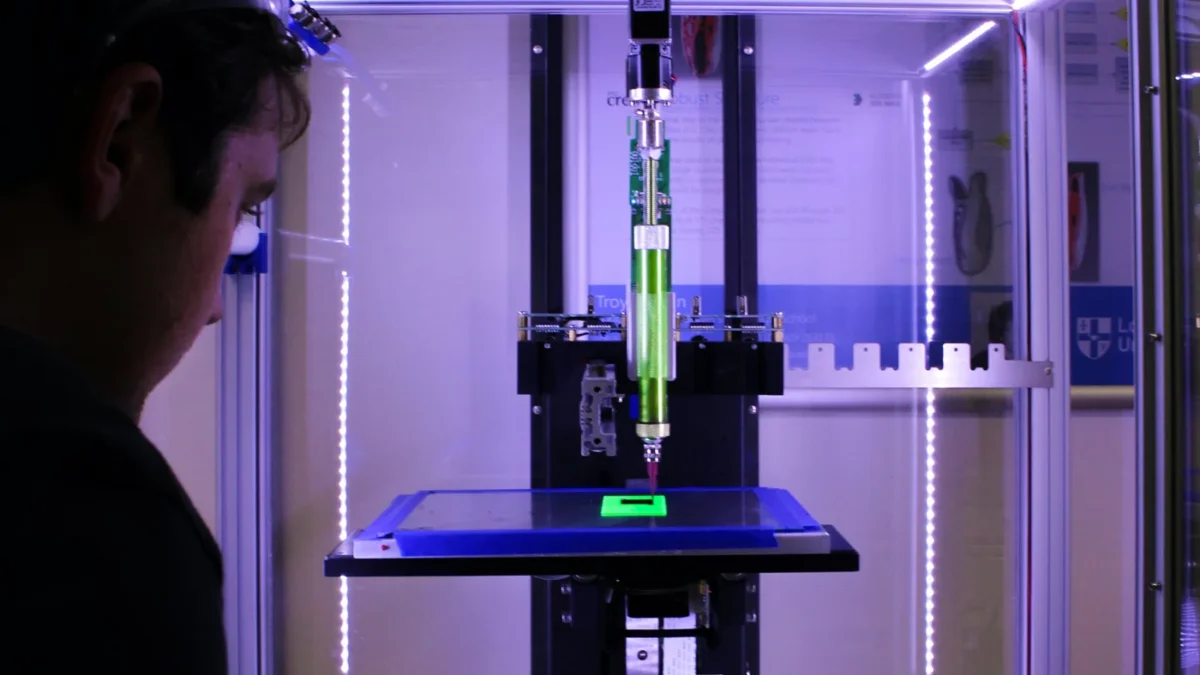
Visual Inspection for Quality Control
Traditionally visual inspection has been applied in various industries for the sake of ensuring product consistency and avoiding defects at the end of the production line and cycle. In batch and discrete industries such as food and pharmaceuticals the inspections are often mandatory due to strict regulations on the products to ensure consumer health and safety. However, in industries such as semiconductors, automotive, defense and materials the cost of defects and the complexity of retrofitting is driving the need for visual inspection to guarantee quality assurance along the production line.
Several methodologies have been created to achieve effective and consistent results in these endeavors. These methodologies mainly rely on a human to conduct the inspection, or in some industries hard-coded machine vision algorithms are being utilized.
Visual Inspection Algorithms – A Slow Starter
In industries where visual inspections of products are a necessary part of the process to ensure product safety and quality, manual inspectors have taken the job of ensuring the defect detection. Studies show that only 70% of defects in products are correctly identified, while the remainder are either false positives identified in good products or missed products with defects. This puts a tremendous amount of pressure on both operator and legislation entities, particularly in heavily regulated sectors. These sectors have become pioneers utilizing computer vision technology to improve their visual inspection capabilities.
As part of the effort to systemize and enable digitally enhanced quality control, early adopter sectors utilized internal and third-party process experts responsible for developing algorithms to structure and define product defects. These algorithms, with aid of early high-speed cameras inline, enable the creation of machine vision for product quality control. While innovative, the impact of this technology has been relatively small due to some of its limitations in the execution stage. The algorithm development relied on expert knowledge to transfer the product quality know-how into machine code and this process is extremely time-intensive for many pioneering sectors.
Another downfall of this legacy system was the required reconfiguration every time a new product or new feature is added to the process line. This resulted in non-scalable returns and high maintenance cost for industries where packaging changes and new product launches were typical. The primitive vision systems failed to be adopted by adjacent markets and all industry players due to their fundamental flow preventing them from easily being implemented and providing adaptability for a long period of time.
Value of Artificial Intelligence in Visual Inspection
Over the past 5 years, collective knowledge and theories around artificial intelligence (AI) have been formulated around the conditions of manufacturing and industrial realities. These new types of self-learning and trainable algorithms are proving to be powerful allies in the path to automize and digitalize visual inspection in multiple industrial segments. Many software platforms and data models have emerged as AI rises to power in the agendas of the executives. One algorithm that mimics the human brain morphology is posing the most potential for visual inspection tasks. This deep learning-based neural network that enables faster and more accurate recognition of images and live feeds through rigorous training with sample visual or video sets.
The neural network-based models prove to be much more accurate and faster than manual inspectors while providing a trail of their records automatically. The only requirement to enable these powerful engines is to train them with an equal amount of “good” and “defected” products. This training requirement has proven tough for several industrial players, but the ones that have already constructed data libraries for defects and product images have quickly trained and implemented the systems in their operations.
The new deep learning driven machine vision systems alleviate one major barrier of legacy ones: The need for a product expert to configure the algorithm. This training and self-learning feature of the deep learning algorithms massively reduces the time to implement systems in live environments. However, as with every technological advancement when an old problem is solved, new ones arise. The need for a visual library of product defects was a requirement not foreseen by many companies. Only future-proof companies were driven to adopt these algorithms in their operations rapidly. In response, systems to easily capture and structure these types of operational data have quickly emerged in recent years.
However, while conventional deep learning algorithms have provided a solution for one barrier to quality control, there is still a significant to challenge to come to enable wide-spread industry uptake. Market conditions are becoming harder to predict each coming year, with increasing product innovation and range expansion. This poses a threat for conventional deep learning-based systems due to the lengthy training requirements of the model whenever a new product feature or defect is introduced. This characteristic of the methodology creates extra maintenance costs and effort for the organization wanting to maintain the system over a long period of time.
Exciting New Developments in Machine Learning
As the algorithms continue to develop more quickly and more structured data capture emerges, new systems and methodologies have emerged for industrial application. These new systems focus on the data requirement for the training for a range of machine learning systems including but not limited to deep learning algorithms. Automatic machine learning (AutoML) utilizes existing data samples and simulates them in random orders to create virtual copies, essentially expending the available data set to train learning algorithms. This enables companies with limited access to data to implement and train machine learning algorithms, which also is true for deep learning algorithms.
To alleviate the pressure of adaptability, a new type of deep learning algorithm is emerging for industrial use cases. Lifelong Deep Neural Networks (L-DNN), aim to reduce the training requirement of neural networks by enabling training for new feature or defects on the fly. This prevents the necessity of going through complete cycles of training whenever a new product or feature is introduced into the system. This is accomplished with L-DNN by focusing on the “good” features from the get-go so that new defect definitions do not impact the system’s capability to assess and categorize product quality.
Industrial Opportunities
As the markets settle and prepare for the normalized state post-COVID, industries realize the benefits and feasibility of new technologies to augment their operations. Visual inspection has been at the center of operational excellence efforts for decades, and new tools and methodologies are reducing the cost of solutions and expertise. This poses an opportunity for a wide array of industrial companies from different sectors to reap the benefits of reliable, flexible, and automated visual inspection in their processes.
However, with new opportunities for innovative organisations come threats for incumbents. The new age of deep learning algorithms will shape the future of manufacturing where digitalized industries will prosper, while laggards will face the consequences of failing to keep up more drastically than ever before.