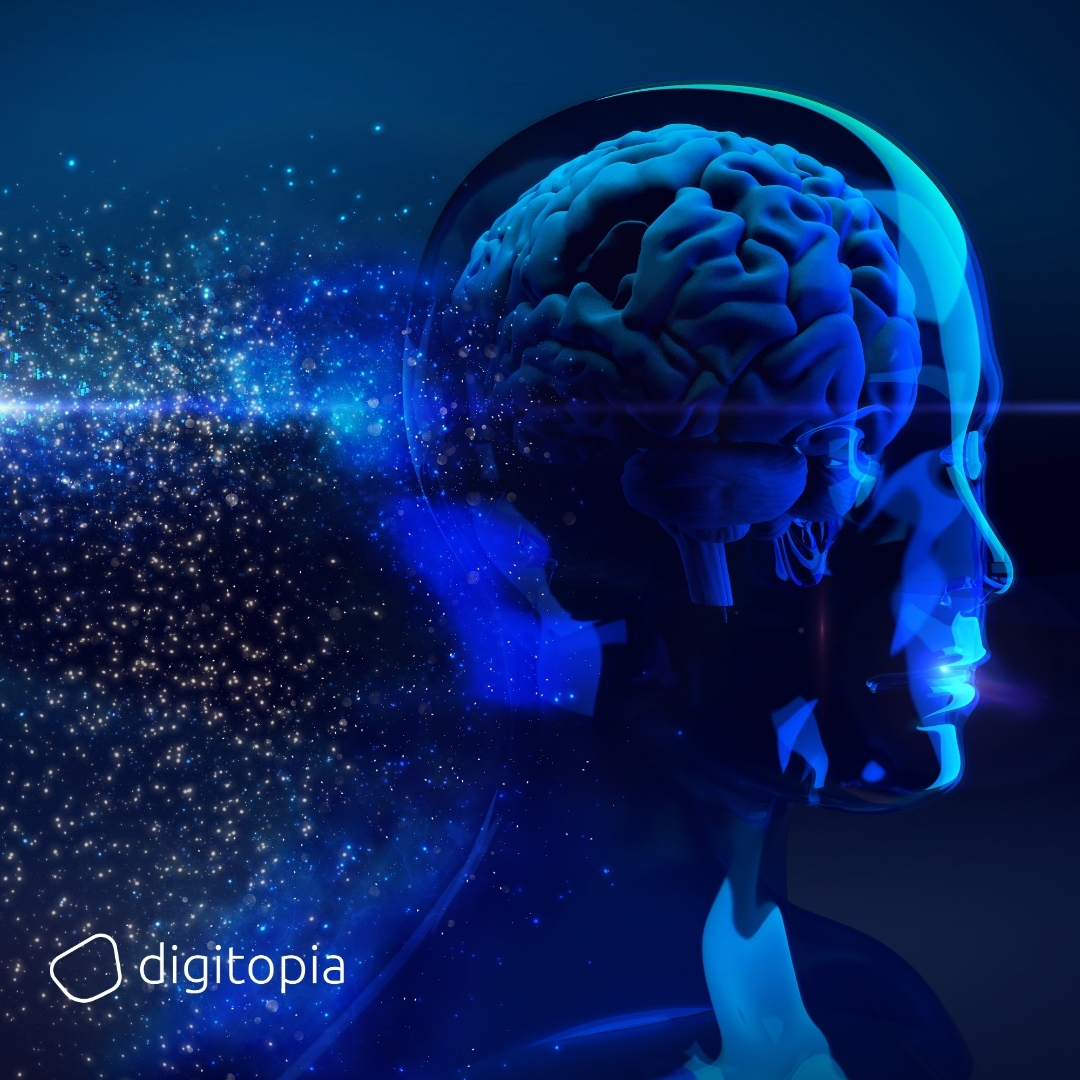
Artificial Intelligence (AI) is no longer a niche discipline reserved for Silicon Valley startups and academic labs. It has become a ubiquitous force reshaping business models, disrupting global industries, and redefining how humans interact with machines. From automating mundane tasks to providing deep insights for strategic decisions, AI has evolved into an indispensable driver of innovation, efficiency, and personalization. Its impact extends far beyond technology—touching healthcare, transportation, finance, education, governance, and beyond.
In this expanded article, we take a deep dive into the artificial intelligence applications, exploring its evolution, the key trends fueling its rapid growth, real-world use cases, pioneering companies and startups, influential voices, and plausible future scenarios. Whether you’re an executive shaping digital transformation or a curious observer seeking insights into tomorrow’s possibilities, a comprehensive understanding of AI is now essential.
Key Trends and Developments in Artificial Intelligence
The AI landscape is evolving at breakneck speed, powered by breakthroughs in deep learning, increased computational power, and the steady accumulation of massive datasets. Yet it’s not just about technological leaps—growing concerns around ethics, regulation, and transparency also shape how AI tools are designed, deployed, and governed. Below are some of the most influential trends redefining AI today.
1. Foundation Models and Large-Scale AI
Originally popularized by natural language processing models such as GPT (Generative Pre-trained Transformer), foundation models learn from vast amounts of data to handle a wide range of tasks. Whether drafting legal documents, analyzing code, or writing creative content, these models demonstrate generalized capabilities previously considered a hallmark of human intelligence. Companies like OpenAI, Google, and Meta invest heavily in refining these architectures, driving progress in language understanding, image generation, and even game-playing AI systems.
2. Edge AI and On-Device Intelligence
As devices proliferate and data volumes explode, processing information at the source becomes increasingly appealing. Edge AI—the practice of running AI algorithms locally on devices—reduces latency and ensures greater privacy by avoiding the need to send data to the cloud. This shift unlocks new possibilities in real-time analytics, augmented reality, robotics, and personalized user experiences, vital for industries like healthcare (portable diagnostic devices), automotive (driver-assist systems), and retail (smart checkout counters).
3. Explainable and Responsible AI
In healthcare, finance, and law enforcement, AI decisions can have life-altering consequences. Hence, there’s a growing insistence on transparency, fairness, and accountability in AI systems. Tools and techniques to interpret model decisions—often referred to as explainable AI—help stakeholders understand how an algorithm arrives at specific conclusions. This is critical for building trust in AI-driven decisions and ensuring they comply with legal and ethical standards.
4. AI for Climate and Sustainability
Amid intensifying climate concerns, AI-driven methodologies for resource optimization, emission monitoring, supply chain efficiency, and renewable energy management are gaining traction. AI can help companies track carbon footprints, manage resources more judiciously, and identify inefficiencies that contribute to environmental degradation. Governments and NGOs also rely on AI to improve disaster preparedness, map deforestation, and accelerate clean energy transitions.
5. AI Ethics and Regulation
From the General Data Protection Regulation (GDPR) in the European Union to proposed guidelines in the United States, lawmakers and regulatory bodies globally are grappling with how to govern AI. Issues such as algorithmic bias, privacy, data protection, and autonomous decision-making demand robust oversight. The ways in which these regulations evolve will deeply influence AI’s trajectory, especially regarding sensitive applications like facial recognition, predictive policing, and healthcare diagnostics.
Practical and Visionary Use Cases
AI is no longer confined to science fiction—it’s a business-critical tool with wide-ranging applications across industries. This section highlights concrete examples of how AI is making an impact today, as well as visionary concepts that foreshadow a more interconnected and intelligent future.
1. Healthcare and Diagnostics
From early cancer detection to personalized treatment plans, AI’s ability to analyze complex medical data has revolutionized healthcare. Startups like Tempus and Paige are applying machine learning to identify genetic markers for disease. Meanwhile, AI-assisted robotic surgery systems, such as those developed by Intuitive Surgical, reduce the margin of error and accelerate patient recovery times. The future might bring fully AI-driven drug discovery pipelines, drastically cutting R&D costs and timelines.
2. Autonomous Transportation
Self-driving technologies rely on real-time AI processing for perception, mapping, and decision-making. In addition to well-known car pioneers like Tesla and Waymo, companies like TuSimple (trucking) and Volocopter (air taxis) demonstrate that autonomous vehicles aren’t limited to personal transport. With advanced machine vision and sensor fusion, driverless logistics fleets can reduce shipping costs, increase safety, and reshape entire industries.
3. Financial Services
Robo-advisors automate personalized investment strategies, while fraud detection systems scan millions of transactions to spot anomalies. Firms like Kasisto and Personetics use AI to enable conversational banking, improving customer engagement. Meanwhile, high-frequency trading outfits increasingly rely on reinforcement learning algorithms to make split-second decisions in dynamic markets. Blockchain-based AI platforms might emerge, blending decentralized finance with intelligent analytics.
4. Manufacturing and Supply Chain
Robotics, computer vision, and predictive analytics collaborate to optimize production lines. For instance, AI-driven systems at Siemens predict machinery malfunctions before they occur, minimizing downtime. In warehouses, Amazon Robotics picks and packs products autonomously, guided by AI that continually refines pathfinding and prioritization. Looking ahead, fully automated “lights-out” factories could run 24/7 with minimal human intervention, drastically reducing labor and operational costs.
5. Creativity and Content Generation
In advertising, AI copywriters such as Jasper or Copy.ai generate everything from social media posts to product descriptions. Musicians collaborate with AI tools to compose novel melodies, while filmmakers experiment with AI for script drafting and special effects. Generative Adversarial Networks (GANs) power realistic deepfake technologies, raising questions about authenticity and intellectual property rights. The conversation around creativity, ownership, and ethical boundaries is becoming increasingly urgent.
Leading Companies in AI
A diverse array of organizations is shaping AI’s evolution. Tech giants leverage their vast resources and data pools to drive foundational research, while more specialized enterprises provide sector-specific AI solutions. This overview spotlight key players forging the path.
Google (Alphabet)
Through innovations like TensorFlow, Bard, and Google Brain, Alphabet remains at the forefront of AI research and commercial application. Its focus areas span language models, image recognition, and quantum computing hybrids.
OpenAI
Initially founded as a nonprofit research lab, OpenAI has championed large-language models (GPT series), reinforcement learning breakthroughs, and commercial AI APIs. Its collaborations with Microsoft bolster AI integration within cloud services.
IBM
IBM’s Watson platform democratized enterprise AI, now focusing on industry-specific verticals such as Watson Health and Watson Finance. Emphasis on trust, explainability, and compliance underpins the company’s corporate AI vision.
Microsoft
From Azure Cognitive Services to the GitHub Copilot developer tool, Microsoft invests in AI solutions across cloud computing, productivity software, and business analytics. Its strategic partnerships with OpenAI signal deeper, long-term commitments to AI.
Baidu
One of China’s tech titans, Baidu is known for natural language processing, AI chips, and autonomous vehicles. Its research labs push innovations in voice assistants, large-scale language models, and AI cloud platforms.
Nvidia
A hardware powerhouse, Nvidia’s GPUs are indispensable for training deep neural networks. The company also offers software stacks, such as CUDA and cuDNN, enabling developers to harness AI at scale.
Additional notable mentions include Amazon (AWS Machine Learning), Meta (previously Facebook, investing heavily in AI for social platforms and metaverse visions), and Oracle (integrating AI with enterprise resource planning and analytics).
Emerging Startups to Watch
While industry giants set the pace, nimble startups often provide disruptive insights, novel architectures, or specialized solutions that can rapidly scale. Below are some up-and-coming companies pushing AI boundaries.
Hugging Face: Originally an NLP-focused startup offering user-friendly transformers libraries, it has grown into a central open-source hub for model development, hosting, and community-driven AI innovation.
Anthropic: Founded by ex-OpenAI researchers, Anthropic explores large-language models, ethical guardrails, and alignment research to ensure AI systems act beneficially.
Graphcore: Developing the Intelligence Processing Unit (IPU), Graphcore promises performance gains in training and inference, supporting complex workloads in natural language understanding and recommendation systems.
Tecton: Specializing in feature stores for machine learning, Tecton aims to streamline MLOps by simplifying how data is processed, stored, and accessed for AI models.
Creyon Bio: Bridging synthetic biology and computational modeling, Creyon Bio leverages AI to accelerate drug discovery and optimize genetic engineering.
Other startups worth noting include DataRobot (automated machine learning), SambaNova (reconfigurable AI hardware), and Cohere (NLP models for enterprise). Each addresses a different pain point within AI’s rapidly expanding ecosystem.
Quotes from Visionary Leaders
Influential voices from tech, academia, and policy shape public discourse around AI. Here, we capture insights from key figures framing the future of human-computer collaboration.
“AI is probably the most important technology that humanity has ever worked on.”
— Sundar Pichai, CEO of Alphabet (Google)
“In the long term, AI and automation are going to be taking over so much of what gives humans a feeling of purpose.”
— Elon Musk, CEO of Tesla and SpaceX
“Smart automation using AI and robotics is the way forward. We need to figure out how to best integrate this into a society that benefits everyone.”
— Satya Nadella, CEO of Microsoft
“We must move AI from the realm of hype to the realm of responsible transformation, or risk losing the greatest opportunity of our generation.”
— Fei-Fei Li, Co-Director of Stanford Institute for Human-Centered AI
Leading Institutions Driving AI Research
Academic labs and research-focused organizations worldwide remain pivotal in advancing core AI theory, applications, and ethics. Collaborations between universities, government agencies, and private firms are central to sustaining AI’s momentum.
DeepMind (UK): Google’s AI subsidiary gained fame with AlphaGo, AlphaFold (protein folding), and advanced reinforcement learning breakthroughs. Known for tackling fundamental scientific challenges alongside commercial applications.
MIT CSAIL (USA): The Computer Science and Artificial Intelligence Laboratory at MIT has produced seminal work in robotics, natural language, and computational biology. Notable for interdisciplinary collaborations that bridge AI, neuroscience, and ethics.
Stanford University (USA): Home to the Stanford AI Lab, specializing in vision, NLP, robotics, AI ethics, and fairness. Stanford also founded the Human-Centered AI Institute to focus on responsible and inclusive AI development.
Tsinghua University (China): Rapidly ascending global rankings in computer vision, speech recognition, and language models. Collaboration with tech giants like Tencent and Huawei bolsters China’s national AI ambitions.
ETH Zurich (Switzerland): Groundbreaking research in robotics, machine learning theory, and computational neuroscience. ETH fosters spin-offs and patents, bridging academic insight with industrial impact.
Other institutions like Carnegie Mellon University (robotics), UC Berkeley (reinforcement learning, AI ethics), and Oxford’s Future of Humanity Institute (long-term AI risk) significantly enrich AI research worldwide.
Expanded Future Scenarios
AI’s rapid evolution could steer the world toward dramatically different outcomes. Below are three scenarios illustrating potential trajectories—each with its own challenges, opportunities, and ethical considerations.
Pessimistic Scenario: AI-Driven Inequality and Displacement
In this troubling future, AI-induced automation outpaces workforce reskilling, leading to widespread job losses and social unrest. A handful of tech giants consolidate power, leveraging proprietary algorithms and data to control global markets. Biased algorithms reinforce societal inequalities, sparking class-action lawsuits and complex legal battles. Privacy becomes a luxury, with constant surveillance fueling public distrust and government clampdowns.
Optimistic Scenario: Collaborative Intelligence for a Better World
In an ideal outcome, AI democratizes access to education, healthcare, and financial services, lifting billions out of poverty. Work becomes more creative and purpose-driven, as AI handles monotonous tasks. Ethical guidelines and global cooperation ensure these systems remain transparent and fair. AI’s predictive power helps mitigate pandemics, optimize agriculture for food security, and reduce resource consumption, fostering a more equitable, sustainable planet.
Realistic Scenario: Gradual, Regulated, and Uneven Adoption
Most industries slowly integrate AI under varying degrees of regulation. Governments impose data protection laws and fairness standards, balancing innovation with oversight. Some jobs are lost, but businesses and policymakers expand retraining programs, helping workers transition. Over time, AI becomes as ubiquitous and taken for granted as the internet—extremely useful yet still prone to gaps in access, compliance issues, and uneven distribution of benefits.
Strategic Implications and Recommendations for Executives
AI’s transformative nature demands deliberate planning. For organizations of all sizes, the journey from experimentation to large-scale AI adoption can be fraught with technical, ethical, and cultural challenges. Here are key strategies for leveraging AI responsibly and effectively.
Start with Clear Use Cases
Rather than attempting to overhaul everything at once, identify specific processes or functions—such as demand forecasting, customer service automation, or predictive maintenance—where AI can deliver tangible ROI.
Build Cross-Functional AI Teams
AI thrives at the intersection of domain expertise, data science, and software engineering. Enable fluid collaboration among R&D, IT, and business units to foster a data-driven culture.
Prioritize Ethics and Compliance
As AI scales, it comes under increased scrutiny for bias, privacy, and fairness. Adopt frameworks like the EU’s AI Act or NIST guidelines to ensure compliance, and measure success through fairness metrics, transparency reports, and accountability audits.
Invest in Infrastructure and Talent
High-performance computing resources and skilled professionals are prerequisites for scaling AI. Competitive salaries and continuous upskilling help attract and retain data engineers, AI researchers, and MLOps specialists.
Prepare for Continuous Change
AI evolves at a faster pace than most other technologies. Maintain agility by incorporating new advancements in deep learning, edge AI, or quantum computing. Periodic technology audits and pilot programs can keep you ahead of the curve.
AI as a Catalyst for Transformative Change
Artificial intelligence has emerged as more than a technological novelty—it is a force shaping our economies, social structures, and individual choices. Its potential to automate, augment, and accelerate human endeavors is immense, but its pitfalls—bias, surveillance, and displacement—are equally significant. Executives, policymakers, and consumers alike bear collective responsibility for directing AI’s power toward ethical, inclusive, and sustainable ends.
For businesses, this means crafting a forward-thinking AI strategy, investing in robust data ecosystems, and fostering a culture of continuous learning. For societies, it requires inclusive dialogues, proactive policymaking, and a commitment to bridging digital divides. Embracing AI doesn’t equate to relinquishing human agency—it underscores our ability to collaborate with intelligent systems to solve humanity’s most pressing challenges, from healthcare disparities to climate change.
As we stand on the cusp of an AI-driven era, the question isn’t whether AI will transform our world—but rather how we can collectively shape that transformation for the better.