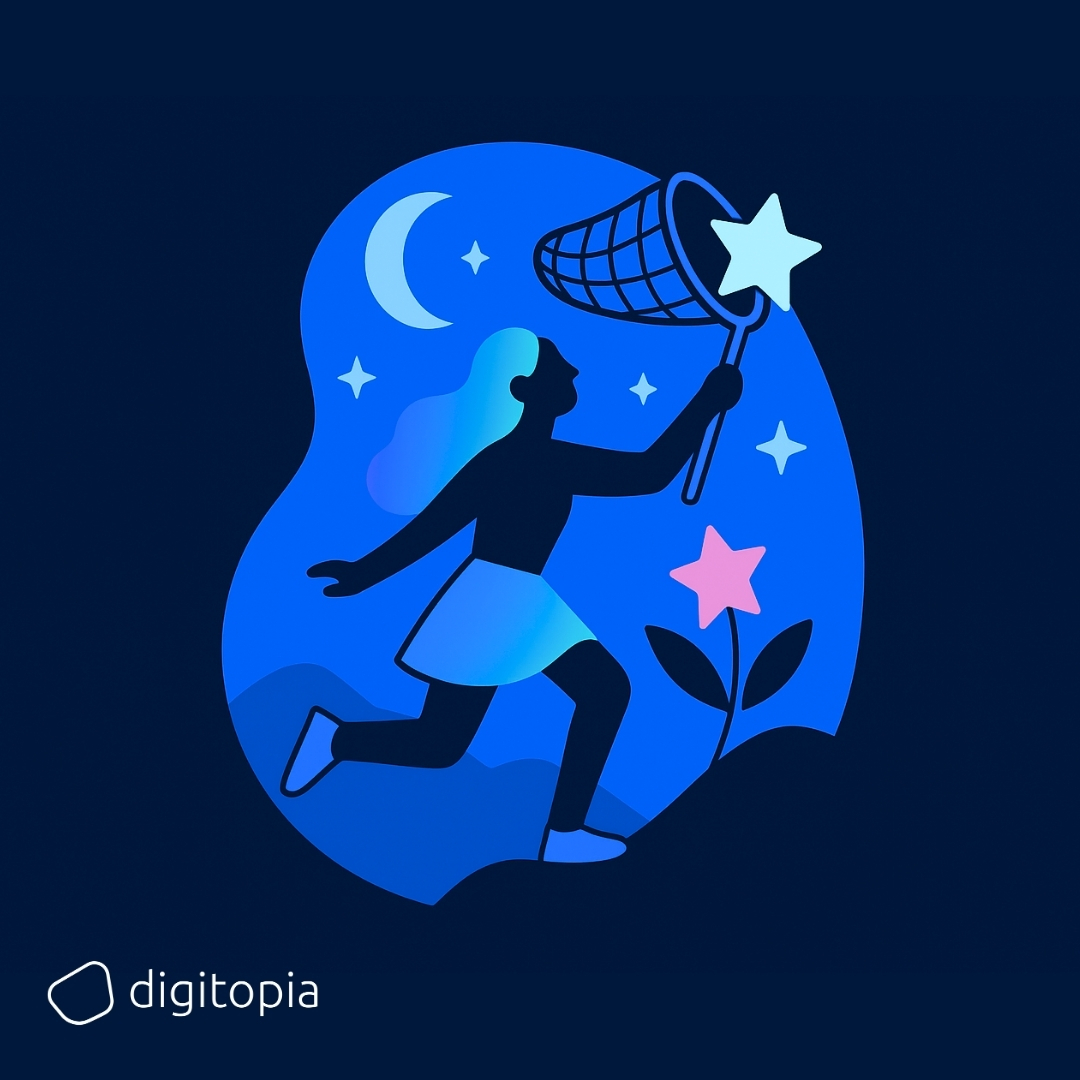
Rebecca Chang stepped into the boardroom feeling both excited and uneasy. As the newly appointed Director of Data Analytics for a multinational retail conglomerate, she had been entrusted with a monumental task: modernize the company’s entire analytics ecosystem. The project involved migrating outdated data warehouses to cloud-based platforms, revamping reporting dashboards used by thousands of employees, and introducing machine-learning models to predict consumer trends. Yet in the swirl of technical details and organizational expectations, Rebecca sensed that delivering on these goals required more than advanced data skills. The key, she suspected, lay in building trust, forging collaborative ties, and uniting diverse teams behind a shared vision.
At first glance, the challenges appeared to be purely technical—establishing pipelines, consolidating records, and ensuring robust governance. But beneath the surface, Rebecca saw competing business requirements and pockets of skepticism. Would the sales division accept new forecasting tools? How might the compliance department react to cloud-based solutions? And crucially, would top executives grant the financial and cultural support needed to sustain such a sweeping transformation? These questions underscored that the real barrier wasn’t merely code or infrastructure; it was alignment among people with different priorities, all of whom needed to become eager partners in an analytics revolution.
The Tension of Modern Data Leadership
In an era where data is touted as the “new oil,” companies scramble to leverage analytics for everything from consumer personalization to supply-chain optimization. Yet data leaders often find themselves at the intersection of high-level strategy and day-to-day execution. On one hand, executives demand immediate returns and sensational dashboards that promise swift insights; on the other, data teams juggle technical complexities, legacy systems, and departmental silos.
The tension is compounded by the speed of innovation. Just as an organization adopts a particular data platform, a new cloud service or AI technique emerges that threatens to render existing approaches obsolete. Meanwhile, employees in finance or merchandising might resent having to learn yet another analytics tool, or fear that automation will make their roles redundant. In this environment, a data leader’s success hinges not just on setting up the right architecture, but on acting as a bridge-builder—one who harnesses empathy, communication, and strategic thinking to unify people around a common goal.
This is precisely the role Rebecca found herself embracing. Although her background in data engineering and predictive modeling provided the foundation, it was her aptitude for navigating human dynamics that allowed her to push the analytics agenda forward without alienating the very people who would determine its success.
Rebecca’s Early Steps: Listening and Discovery
From the moment she accepted her new role, Rebecca committed to spending her first month listening rather than imposing a solution. She scheduled one-on-one meetings with department heads across marketing, finance, operations, legal, and compliance. Rather than starting with any grand pronouncement, she opened these sessions with questions:
“What data do you rely on most, and why?”
“Which reports or dashboards give you headaches?”
“If you could magically solve one analytics challenge, what would it be?”
These conversations revealed pain points that weren’t strictly about technology. In marketing, managers lamented that monthly campaigns were hampered by slow data refresh cycles, forcing them to make gut decisions. Finance teams worried that the risk of inaccurate data might lead to regulatory fines. Operations wanted real-time dashboards to track inventory but struggled with a patchwork of disconnected systems. Meanwhile, compliance needed assurances that sensitive consumer data would remain properly secured in any new architecture.
Rebecca also discovered cultural frictions. Some departments felt overlooked in budget discussions, fearing their analytics requests were always deprioritized in favor of flashy AI pilots that never seemed to reach maturity. Others questioned the cost-effectiveness of switching to the cloud, citing fiascos at competitor firms that had experienced massive outages or security breaches.
Rather than dismiss these concerns, Rebecca captured them meticulously in a comprehensive “data empathy map.” She noted each group’s objectives, fears, and suggestions. This map became her guiding reference, reminding her that a solution might be technically elegant but would fail if it ignored the nuanced needs of various stakeholders.
Defining a Shared Vision
One of Rebecca’s core beliefs was that a transformation would stall if it felt like a fragmented patchwork of small projects. Thus, she spent considerable effort articulating a unifying purpose—a narrative explaining why modernizing the analytics ecosystem mattered for everyone, from frontline store managers to top-tier executives. In internal memos and presentations, she framed it as a shift from “reactive guesswork” to “proactive intelligence,” enabling the organization to:
Forecast Demand More Accurately: With better predictive models, teams could avoid stockouts or overstocks, especially during peak shopping seasons.
Refine Customer Engagement: More granular insights into consumer behavior would lead to marketing campaigns that felt timely and relevant.
Streamline Operations: Real-time data could help each department adjust staffing, logistics, and pricing on the fly.
Maintain Trust and Compliance: By consolidating data and auditing usage, the company would safeguard consumer information and minimize regulatory risk.
Rebecca emphasized that these goals were interdependent. Marketing insights hinged on accurate inventory data; finance’s budget projections relied on consistent reporting across departments; compliance demanded comprehensive tracking of who accessed what data and why. Everyone’s success, she argued, was intertwined.
However, she recognized that a lofty vision wouldn’t suffice without tangible milestones. So she proposed a roadmap that spanned 18 months, broken into phases. Early wins, such as automating the daily sales report or rolling out a simplified “insights” dashboard, would demonstrate immediate value. More ambitious AI-driven initiatives, like advanced demand forecasting, were scheduled for later stages, once foundational architecture and data governance were in place. This balance of immediate ROI and long-term innovation helped quell concerns about the dreaded “perpetual pilot syndrome,” where projects lingered in proof-of-concept purgatory without ever scaling.
Building Alliances and Early Adoption
With the roadmap in hand, Rebecca focused on forging alliances. She knew the project’s success hinged on departmental champions—individuals willing to advocate for new analytics tools and workflows. Sometimes, these individuals were formal leaders, such as the Vice President of Marketing. Other times, they were influential “unofficial leaders,” like a senior business analyst who’d been with the firm for 20 years and commanded peer respect.
Rebecca held small workshops with these potential champions, explaining the roadmap in detail and soliciting feedback. She invited them to critique the timeline, suggest alternative tools, and highlight potential pitfalls. The discussions were spirited; some felt the AI portion should start earlier, while others worried about intangible “tech hype” overshadowing practical solutions. Rather than dismiss these viewpoints, Rebecca welcomed them, adjusting minor aspects of the plan where feasible.
By incorporating feedback, she earned a reputation for genuine collaboration. When skepticism surfaced—“But what if this cloud platform goes down?” or “Will the new dashboards slow down my day?”—she provided use cases from other successful transformations within the company’s global network. She also offered pilot programs, letting small teams trial dashboards before rolling them out enterprise-wide.
Perhaps most importantly, she ensured that these champions were recognized. At all-company meetings or in executive briefings, she publicly thanked those who contributed key ideas or identified critical risks early. This culture of shared credit motivated more employees to step forward, fostering an atmosphere of cooperative progress rather than turf wars.
Overcoming Technical and Cultural Hurdles
No transformation proceeds without friction, and Rebecca’s analytics overhaul was no exception. Resistance flared in some quarters. The finance department, for instance, balked at the cost of migrating large datasets to the cloud, pointing to stories of ballooning vendor bills. Meanwhile, a group of store managers resented yet another “corporate-driven tool” forced upon them, doubting it would improve local operations.
Rebecca approached these hurdles with a mix of empathy and evidence. She asked the finance team to map out existing on-prem costs—hardware maintenance, data center cooling, staff hours spent patching servers—and compare them to projected cloud expenses. While the numbers suggested cloud might be more expensive in certain edge cases, the long-term savings in maintenance and the agility to spin resources up or down outweighed initial costs. To assuage store managers, she demoed prototypes that streamlined tasks like daily inventory checks, showing how the new dashboards could reduce manual data entry and free them up for customer-facing activities.
Culturally, she understood that adopting advanced analytics required a learning curve. She budgeted resources not only for technology but also for training. Rather than bury employees in dense manuals, she championed short, engaging training modules that walked users through real scenarios—like adjusting staffing after a sales spike or calibrating marketing spend mid-campaign.
Throughout these conversations, Rebecca intentionally refrained from belittling concerns or hiding negative data. If a pilot revealed that a dashboard loaded too slowly for busy store managers, she acknowledged the flaw and collaborated with the IT team on performance optimizations. If an AI algorithm incorrectly predicted which products would trend next quarter, she framed it as an opportunity to refine the model, not a reason to scrap AI altogether.
Sustaining Momentum Through Governance and Structure
A critical aspect of large-scale analytics transformations is governance—ensuring data quality, security, and consistent usage across a sprawling enterprise. Early in her tenure, Rebecca established a Data Governance Council composed of stakeholders from each major department: marketing, operations, finance, compliance, and IT. This council met monthly to hash out data definitions, set usage policies, and track the progress of analytics initiatives.
By giving each department a seat at the table, the council diffused accusations that “IT just does what it wants.” The group formalized roles—data stewards in each division responsible for vetting the quality of key metrics, for instance—and created a transparent channel to escalate issues like data discrepancies or policy violations. Over time, these governance sessions became a crucible for forging consensus on tricky issues, such as how to anonymize customer records or how to archive historical data to balance performance with cost.
Governance also provided a structured way to measure success. Rebecca insisted that each new analytics project define measurable KPIs—like reduced out-of-stock rates, faster month-end reconciliations, or improved campaign conversion. Periodic reviews helped the council see whether the changes truly delivered on their promises, or if they needed recalibration. These reviews also supplied Rebecca with compelling evidence to share at executive meetings, illustrating how each step in the roadmap contributed tangible value.
Rebecca’s Leadership Style: Empathetic but Decisive
Colleagues often marveled at Rebecca’s ability to juggle so many moving parts—technical issues, user adoption concerns, security policies—while maintaining a sense of calm. Some believed her background in data science predisposed her to logical thinking, but she frequently credited her success to balancing empathy with decisiveness.
Empathy: She never dismissed a worry as trivial, recognizing that user frustration could quickly escalate if left unaddressed. By listening intently, validating fears, and offering solutions, she built goodwill across the organization.
Decisiveness: When a feature or vendor proved underwhelming, she didn’t hesitate to pivot. She was careful to explain the rationale behind such decisions, framing them as learning experiences rather than failures. This blend of transparency and firm direction reassured teams that they weren’t plunging blindly into chaos.
Rebecca’s emotional intelligence also shone in her communication style. While she could geek out on the mathematics of predictive modeling, she tailored her messages to the audience. Executives heard about strategic outcomes and revenue impacts; frontline managers saw how analytics simplified daily tasks. This capacity to “translate” data insights into clear, relatable narratives made her a unifying figure.
Measurable Wins and Lasting Cultural Change
After the first 12 months, the company saw tangible benefits from Rebecca’s analytics transformation:
Improved Forecast Accuracy: Demand forecasting algorithms, fed by real-time sales data, helped reduce stockouts by 15%, boosting customer satisfaction.
Faster Reporting Cycles: Automated data pipelines cut the monthly financial close process by 30%. Finance staff no longer grappled with manual consolidations.
Enhanced Employee Adoption: Over 70% of store managers reported using the new dashboards at least once a day, citing time savings and better decision-making.
Reduced Compliance Risks: Centralized tracking of user data access allowed compliance to pinpoint suspicious log-ins or data anomalies swiftly.
Equally noteworthy were the cultural shifts. Employees across departments grew more comfortable discussing analytics needs and challenges openly. The Data Governance Council evolved into a respected institution, resolving disputes collaboratively instead of descending into finger-pointing. A positive feedback loop emerged: seeing small wins boosted morale, encouraging teams to test further possibilities, like advanced machine learning or deeper personalization.
Rebecca celebrated these wins publicly, from awarding internal “Data Hero” badges to hosting small “Analytics Demo Days,” where employees showcased novel ways they leveraged dashboards. Such gestures reinforced the idea that analytics was everyone’s business, not just IT’s pet project.
Beyond Technical Triumph
Rebecca’s journey highlighted that data transformations aren’t solely about software migrations and advanced algorithms. They revolve around people—how they perceive changes, integrate tools into workflows, and trust the leadership orchestrating it all. Her approach underscored the indispensable role of emotional intelligence in driving adoption, forging unity among stakeholders, and ensuring that technical brilliance translates into day-to-day operational impact.
In her final monthly briefing of the year, she stood before a room of executives who once doubted that the company could transition away from its legacy data silos. “This isn’t the end,” she reminded them, “but the beginning of a more adaptive analytics culture. We’ll keep refining, keep learning, and keep aligning our data initiatives with our core mission: serving our customers better every day.”
The applause that followed wasn’t just for dashboards or AI models; it was for a leadership style that valued open communication, human-centered implementation, and an unflagging commitment to bridging the gap between technology and the people it served.
Nurturing Analytics Success with Empathy
Rebecca Chang’s story illuminates how a single protagonist, armed with empathy and technical insight, can set a mammoth analytics overhaul on a path to success. By beginning with extensive listening, building alliances, and articulating a shared vision, she overcame resistance from skeptical users and budget-conscious departments. Structured governance, tangible milestones, and consistent communication ensured that ambitious AI pilots didn’t overshadow immediate user needs. Finally, her balanced leadership—empathetic yet decisive—anchored the transformation in a culture of trust and collaboration.
For leaders considering or already undertaking a data revolution, her experiences reinforce a core lesson: while robust platforms and sophisticated algorithms provide the backbone, emotional intelligence is the essential connective tissue. Projects may falter if team members don’t see their day-to-day concerns addressed or feel marginalized by a “technology-first” mindset. But when a leader invests the time to understand diverse perspectives, craft an inclusive roadmap, and champion open discussion, the enterprise can unlock analytics excellence that endures well beyond the initial rollout.
This approach resonates far beyond the realm of data analytics. Whether it’s shifting to cloud-based operations, adopting DevOps practices, or implementing cybersecurity measures, empathy, clarity, and decisive leadership can bridge the gap between conceptual breakthroughs and tangible organizational gains. By honoring both the technological complexities and the human realities, a visionary like Rebecca can guide the future of business intelligence—and spark a culture of continuous learning, innovation, and shared success.