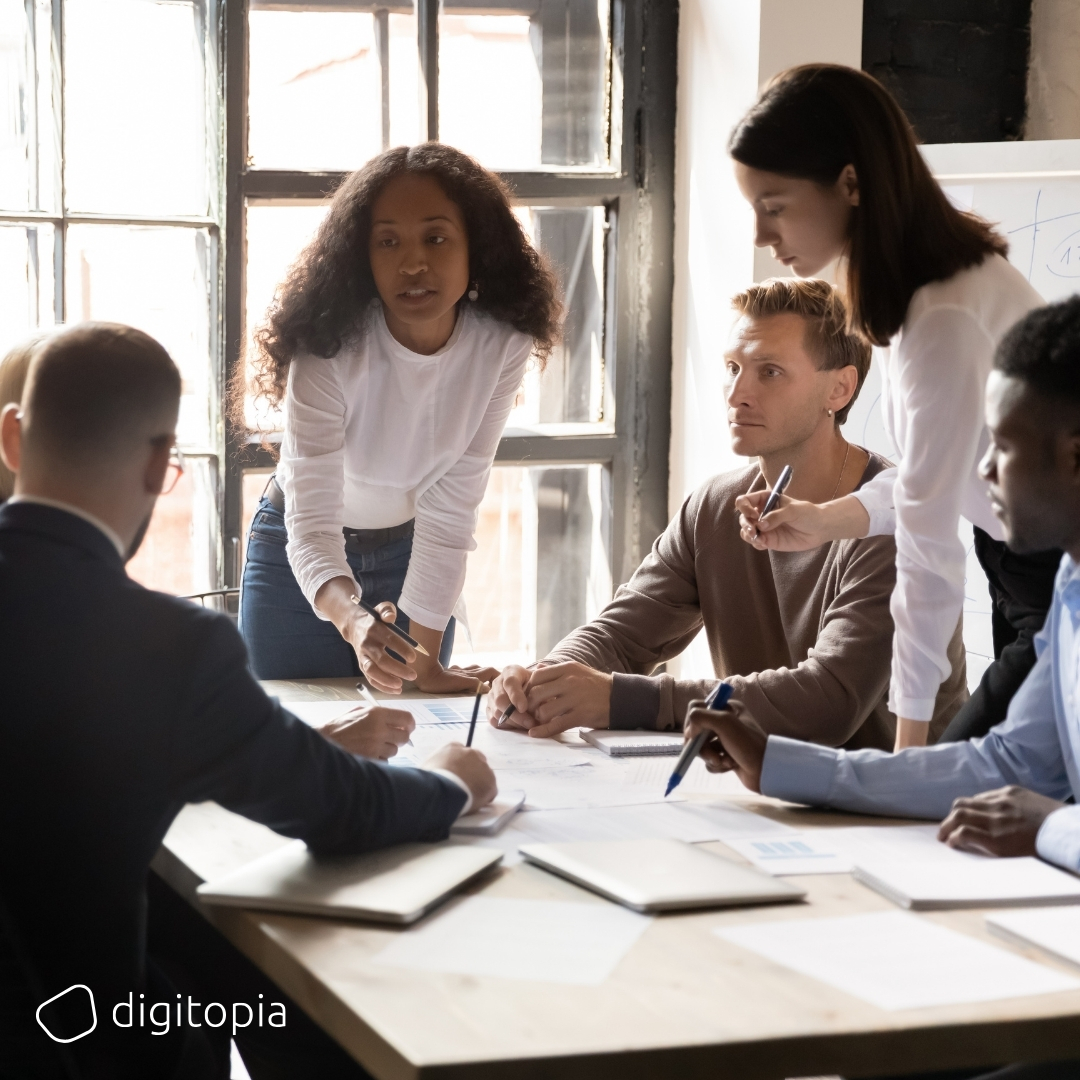
In today’s rapidly evolving business landscape, AI is the new gold rush. Everywhere you look, organizations are touting AI-powered solutions, from chatbots to predictive analytics and autonomous systems. The potential is undeniably alluring: streamline operations, unlock new revenue streams, gain competitive advantage, and revolutionize customer experiences. The hype is real, and so is the fear of missing out (FOMO).
In boardrooms and strategy sessions, leaders feel the pressure to jump on the AI bandwagon. The belief is clear: if you’re not investing in AI, you risk being left behind. As a result, companies are pouring millions into AI projects, hiring data scientists, building data lakes, and rushing to demonstrate how they are embracing this transformative technology. However, there’s a harsh reality beneath the surface. Despite the buzz, many AI initiatives fail to deliver tangible business outcomes.
Why is this happening? Because AI isn’t magic—it’s science, engineering, and a strategic discipline. Rushing into AI projects without a clear understanding of their complexities often leads to disillusionment. The challenges range from poor data quality and lack of infrastructure to insufficient buy-in from stakeholders and an inability to scale. The truth is, AI projects are fraught with pitfalls, and navigating them requires more than enthusiasm and FOMO-driven urgency. It demands preparation, expertise, and a clear alignment of technology with business goals.
In this blog post, we’ll explore the 5 most common ways organizations mess up their AI projects, uncover the root causes, and provide actionable recommendations to ensure your AI investments deliver real value. Whether you’re a business executive, project leader, or data scientist, this guide will help you avoid costly mistakes and turn your AI ambitions into lasting success.
1. Believing That the Proof of Concept (PoC) Will Work in Production:
AI PoCs are exciting; they demonstrate potential and generate buy-in. However, success in a controlled PoC environment often doesn’t translate directly to production.
Main Reasons for Messing Up
- Over-optimized for PoC environments: PoCs often run with clean, limited data and controlled variables, unlike messy, real-world conditions.
- Lack of planning for production challenges: Deployment, monitoring, and scaling are often ignored during PoC stages.
Key Recommendations
- Build with the end in mind: Ensure the PoC is designed with production scalability, data flow, and real-world variability in mind.
- Plan for production from day one: Include infrastructure, compliance, and user feedback in PoC planning.
Case Study: A retail company developed an AI-driven demand forecasting tool in a PoC using historical data. It achieved 90% accuracy in the PoC but failed miserably in production because live data contained outliers and inconsistencies that weren’t accounted for. A redesign incorporating real-time anomaly detection salvaged the project.
2. Not Being Able to Scale the Infrastructure:
AI projects often stall when scaling from prototypes to enterprise-wide deployments due to inadequate infrastructure.
Main Reasons for Messing Up
- Underestimating compute and storage needs: Training large models requires significant resources that may not have been accounted for.
- Lack of cloud strategy: On-premises infrastructure often fails to meet the dynamic needs of AI scalability.
Key Recommendations
- Adopt cloud-native AI platforms: Use elastic compute resources for training and deployment.
- Plan for growth: Design the architecture to handle increasing data and complexity over time.
Case Study: A mid-sized healthcare company developed an AI-based patient diagnosis system. Initial success gave way to frustration when the system couldn’t handle the sudden influx of real-time queries. Migrating to a cloud platform enabled seamless scaling and improved response times.
3. Top Talent Leaves in the Middle of the Project:
AI talent is in high demand, and losing key personnel mid-project can disrupt timelines and knowledge continuity.
Main Reasons for Messing Up
- Poor project management: Delays and unclear objectives frustrate employees.
- Lack of engagement: Talented AI professionals often leave for more challenging, rewarding opportunities.
Key Recommendations
- Create a compelling vision: Keep your team motivated with a strong purpose and meaningful work.
- Invest in retention strategies: Offer competitive pay, career development opportunities, and an engaging workplace culture.
- Document thoroughly: Use knowledge management tools to ensure continuity.
Case Study: A financial services firm lost its lead data scientist halfway through developing a fraud detection system. By implementing mentorship programs and offering bonuses for project completion, they retained the rest of the team and successfully delivered the project.
Struggling with AI project challenges?
Discover where you stand with the AI Maturity Index and take actionable steps to drive real business value. AIMI helps organizations assess their AI readiness, identify gaps, and build a strategic roadmap for successful AI implementation.
4. Underestimating Data Quality and Data Engineering:
AI is only as good as the data feeding it. Poor-quality data leads to inaccurate predictions, biased outputs, and failed deployments.
Main Reasons for Messing Up
- Ignoring data preparation: Data cleansing and preprocessing are often rushed or overlooked.
- Lack of a robust data pipeline: Data inconsistencies and bottlenecks hamper AI performance.
Key Recommendations
- Prioritize data engineering: Invest in robust ETL (extract, transform, load) pipelines.
- Focus on data governance: Ensure data quality, compliance, and lineage are part of your strategy.
- Iterate and validate: Continuously refine data models to adapt to evolving inputs.
Case Study: An e-commerce company’s recommendation engine failed due to duplicate and incomplete data entries. After hiring a dedicated data engineering team and establishing governance protocols, the system achieved 30% higher click-through rates.
5. Nobody Appreciates AI’s Results and Capabilities:
Even the most advanced AI projects fail if their value isn’t understood or embraced by end users and decision-makers.
Main Reasons for Messing Up
- Poor change management: Users resist adopting AI tools when they don’t understand how they add value.
- Lack of explainability: Complex models often produce results that are hard to interpret or trust.
Key Recommendations
- Engage stakeholders early: Involve end users, managers, and decision-makers in project development.
- Focus on explainable AI: Design models with transparency and interpretability in mind.
- Demonstrate value: Quantify the impact of AI solutions through measurable outcomes.
Case Study: A manufacturing firm developed a predictive maintenance solution, but operators ignored the AI alerts because they didn’t trust the system. By integrating visual dashboards and explaining the predictions, trust improved, reducing downtime by 20%.
Final Thoughts
AI projects are complex endeavors that require careful planning, execution, and communication. Avoiding these common pitfalls can mean the difference between failure and transformative success.
Recap of Key Recommendations
- Build PoCs with production in mind.
- Scale infrastructure using cloud-native tools.
- Retain talent with meaningful projects and competitive benefits.
- Prioritize data quality, governance, and engineering.
- Involve stakeholders and emphasize AI’s explainability.
By learning from these lessons and applying these strategies, your AI project can move from proof of concept to delivering measurable business value seamlessly.