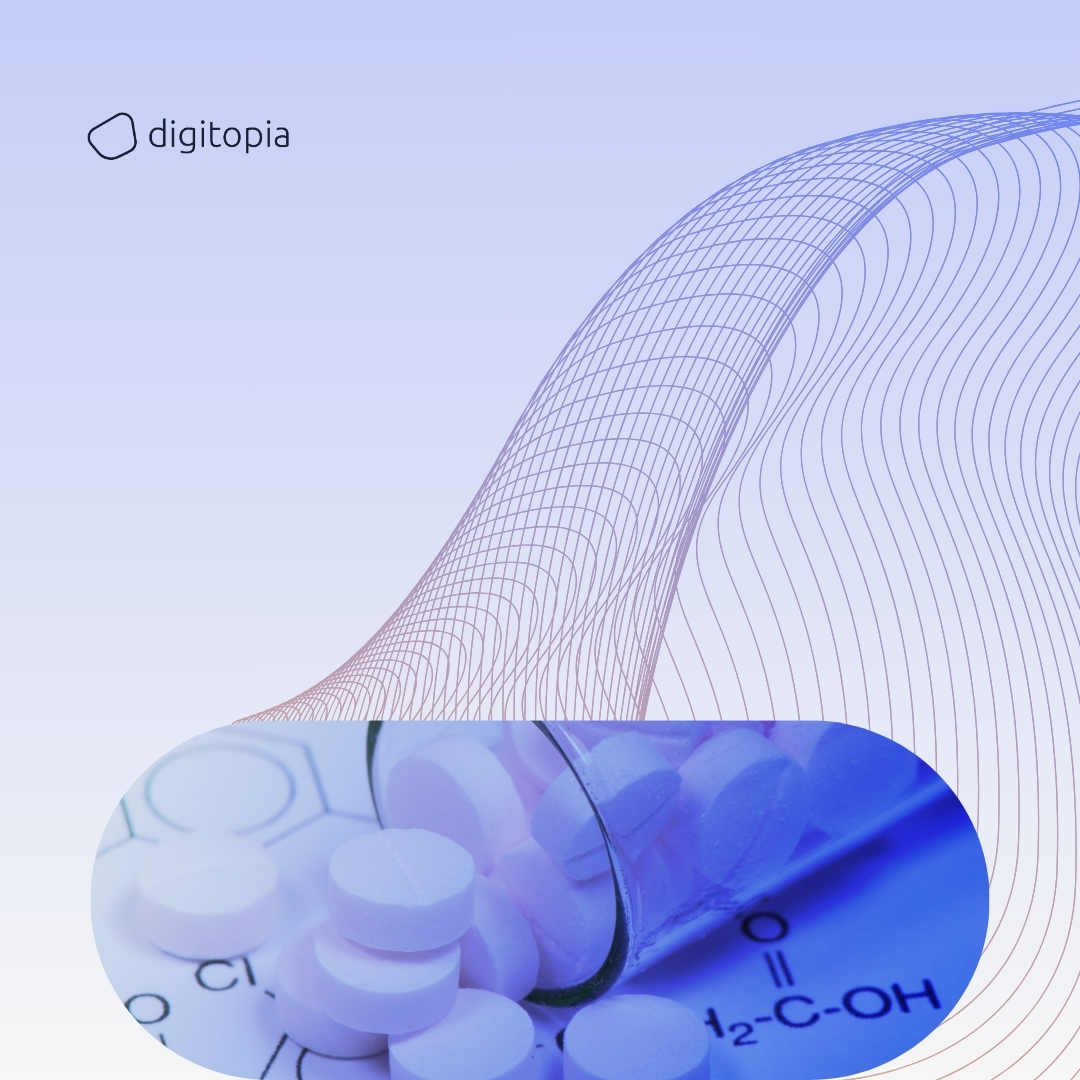
The pharmaceuticals industry is experiencing a transformative shift driven by Artificial Intelligence (AI). From drug discovery and development to personalized medicine and supply chain optimization, AI is revolutionizing various aspects of the pharmaceutical sector. Discover the pivotal role of AI in pharmaceuticals industry, exploring key use cases that accelerate drug discovery, enhance precision in research, and streamline development processes.
The Promise of AI in the Pharmaceuticals Industry
AI holds immense potential to drive innovation and efficiency in the pharmaceuticals industry. By leveraging AI technologies, pharmaceutical companies can accelerate drug discovery, optimize clinical trials, personalize treatment plans, and streamline supply chain operations. The integration of AI into pharmaceutical processes not only reduces costs and time-to-market but also improves patient outcomes and safety. Embracing AI is essential for pharmaceutical companies aiming to stay competitive in a rapidly evolving healthcare landscape.
Learn More: Top 10 Secrets to Succeeding with AI
Our comprehensive ebook provides actionable insights, real-world case studies, and proven strategies to help you integrate AI into your operations and drive growth.
Download Now
Key Use Cases in the Pharmaceuticals Industry
AI is revolutionizing the pharmaceuticals industry by accelerating drug discovery, optimizing clinical trials, personalizing medicine, and streamlining supply chain operations. By harnessing the power of AI, pharmaceutical companies can significantly reduce the time and cost of bringing new drugs to market, improve patient outcomes, and enhance operational efficiency. This section explores the most impactful AI use cases in the pharmaceuticals industry, demonstrating how these technologies are driving innovation and transforming the landscape of healthcare.
Drug Discovery and Development
- Predictive Modeling for Drug Discovery: AI algorithms analyze vast datasets to identify potential drug candidates, predict their efficacy, and optimize molecular structures, significantly reducing the time and cost of drug discovery.
- Virtual Screening: AI-driven virtual screening techniques can quickly evaluate millions of compounds to identify those with the highest likelihood of success in treating specific diseases, streamlining the initial stages of drug development.
- Biomarker Discovery: AI helps identify biomarkers that can predict patient responses to treatments, enabling the development of more targeted and effective therapies.
Clinical Trials
- Patient Recruitment and Retention: AI analyzes patient data to identify suitable candidates for clinical trials and predicts factors that may influence their participation and retention, improving the efficiency and success rate of trials.
- Trial Design Optimization: AI-driven analytics optimize clinical trial designs by simulating various scenarios and identifying the most effective protocols, reducing trial durations and costs.
- Real-Time Monitoring and Analysis: AI monitors clinical trial data in real-time, identifying trends and anomalies that can lead to faster decision-making and more accurate results.
Personalized Medicine
- Genomic Data Analysis: AI analyzes genomic data to identify genetic variations that influence individual responses to treatments, enabling the development of personalized medicine tailored to each patient’s genetic profile.
- Treatment Recommendation Systems: AI-driven systems analyze patient data, including medical history and genetic information, to recommend personalized treatment plans that maximize efficacy and minimize adverse effects.
- Predictive Analytics for Disease Progression: AI predicts disease progression and treatment outcomes based on patient data, helping healthcare providers make informed decisions and adjust treatment plans as needed.
Supply Chain Optimization
- Inventory Management: AI optimizes inventory levels by predicting demand and managing stock efficiently, reducing shortages and excesses in the pharmaceutical supply chain.
- Demand Forecasting: AI-driven demand forecasting models analyze market trends, seasonal variations, and historical data to accurately predict future demand for pharmaceutical products.
- Logistics and Distribution: AI enhances logistics and distribution by optimizing routes, monitoring shipment conditions, and ensuring timely delivery of pharmaceutical products.
Powerful Success Stories in AI-Driven Pharmaceuticals
The AI revolution in the pharmaceuticals industry is already underway, with leading companies demonstrating the transformative power of artificial intelligence. These success stories illustrate the significant benefits of AI adoption, from accelerated drug discovery to enhanced patient care and supply chain efficiency.
Insilico Medicine – Drug Discovery
Challenge: Insilico Medicine, a biotechnology company, aimed to accelerate the drug discovery process and identify promising drug candidates more efficiently.
Solution: Insilico Medicine implemented AI-driven predictive modeling and virtual screening techniques to analyze vast datasets and identify potential drug candidates. Their AI algorithms optimized molecular structures and predicted the efficacy of compounds in treating specific diseases.
Benefits: The AI-driven approach significantly reduced the time and cost of drug discovery for Insilico Medicine. The company identified several promising drug candidates in a fraction of the time required by traditional methods, positioning itself as a leader in AI-powered drug discovery.
Pfizer – Clinical Trials
Challenge: Pfizer needed to improve the efficiency and success rate of its clinical trials, particularly in patient recruitment and trial design.
Solution: Pfizer utilized AI to analyze patient data and identify suitable candidates for clinical trials. AI-driven analytics optimized trial designs by simulating various scenarios and identifying the most effective protocols.
Benefits: The AI-driven approach improved patient recruitment and retention rates, reducing trial durations and costs. Pfizer’s optimized trial designs led to faster decision-making and more accurate results, enhancing the overall success rate of their clinical trials.
Tempus – Personalized Medicine
Challenge: Tempus, a technology company specializing in personalized medicine, aimed to develop treatment plans tailored to each patient’s genetic profile and medical history.
Solution: Tempus implemented AI-driven genomic data analysis and treatment recommendation systems. Their AI algorithms analyzed patient data, including genetic information, to recommend personalized treatment plans that maximize efficacy and minimize adverse effects.
Benefits: The AI-driven approach enabled Tempus to develop highly personalized treatment plans, improving patient outcomes and satisfaction. The company’s innovative use of AI in personalized medicine positioned Tempus as a leader in precision healthcare.
Key Recommendations for Successful AI Transformation in Pharmaceuticals
Successfully implementing AI in the pharmaceuticals industry requires a strategic approach, visionary leadership, and a commitment to continuous improvement. Companies must invest in robust data infrastructures, develop specialized AI expertise, and foster a culture of innovation. By aligning AI initiatives with business goals and leveraging ecosystem partnerships, pharmaceutical companies can drive significant advancements in drug discovery, clinical trials, and patient care. These key recommendations provide a roadmap to harness the full potential of AI, ensuring sustainable growth, enhanced efficiency, and improved patient outcomes in a rapidly evolving healthcare landscape.
- Invest in Data Infrastructure: Develop a robust data infrastructure to collect, integrate, and analyze diverse datasets from various sources across the pharmaceutical value chain. Ensure data quality, security, and accessibility to drive effective AI implementation.
- Develop AI Expertise: Prioritize talent acquisition and development by investing in comprehensive training programs and forming partnerships with educational institutions. Foster a culture of continuous learning and innovation to build a skilled workforce capable of leveraging AI technologies.
- Foster Collaborative Leadership: Leadership teams must champion AI transformation by articulating a clear vision and strategy for AI adoption. Encourage collaboration across departments and functions to ensure alignment and drive AI initiatives forward.
- Leverage Ecosystem Partnerships: Build an ecosystem of partners, including technology providers, academic institutions, and industry consortia, to accelerate AI adoption and innovation. Collaborate with partners for knowledge sharing and access to advanced tools and platforms.
- Align AI Initiatives with Business Goals: Identify and prioritize AI projects that align with strategic business objectives and deliver measurable value. Focus on high-impact use cases that build momentum for broader AI adoption.
- Implement Continuous Measurement and Improvement: Use tools like Digitopia’s AI Maturity Index to continuously assess and benchmark AI capabilities. Regularly review progress, adjust strategies, and ensure AI initiatives deliver the expected value.
By embracing AI across drug discovery, clinical trials, personalized medicine, and supply chain operations, pharmaceutical companies can drive innovation, enhance efficiency, and improve patient outcomes. These advancements will position pharmaceutical companies to lead in a dynamic and rapidly evolving healthcare landscape, ensuring long-term success and resilience.