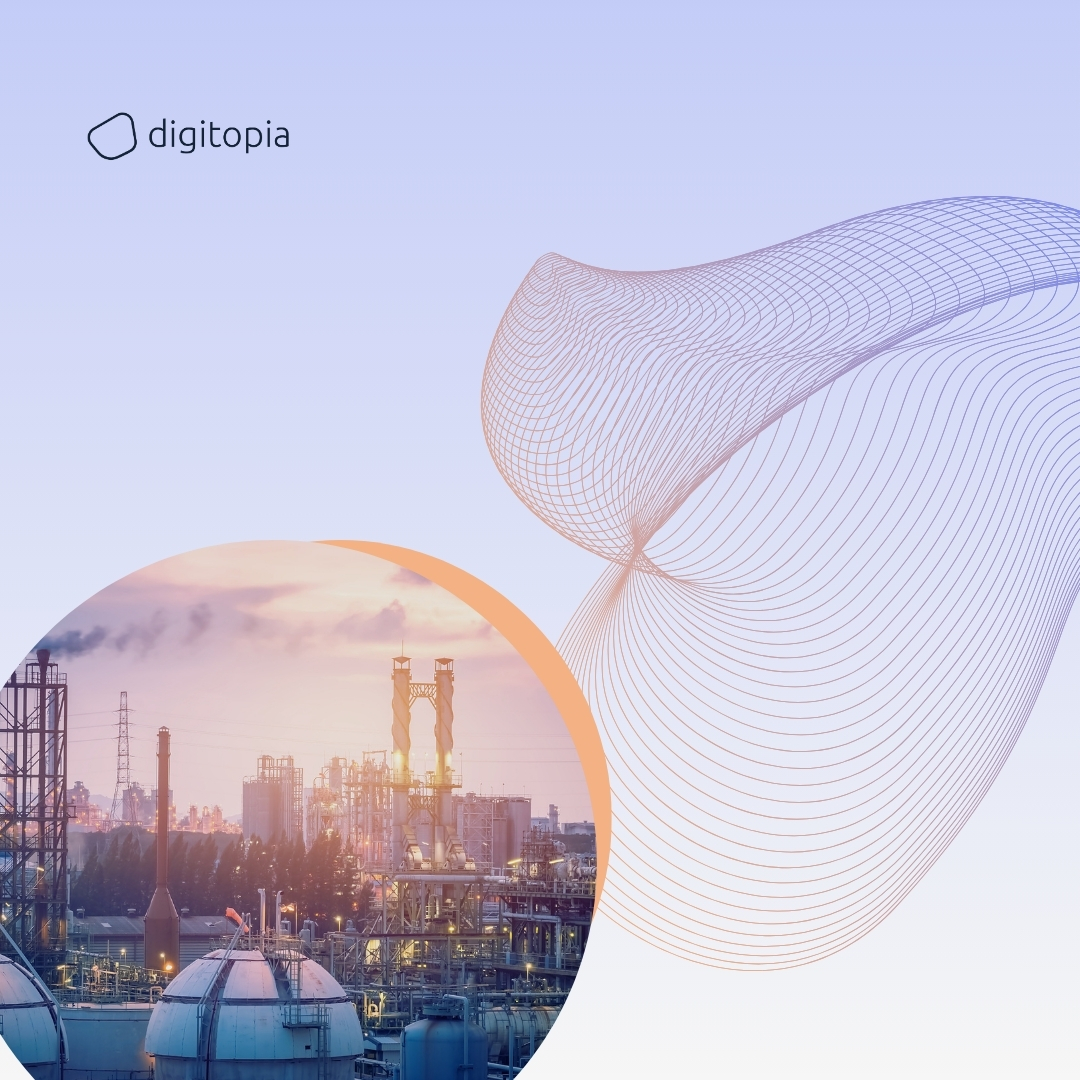
Artificial Intelligence (AI) is poised to revolutionize the manufacturing industry, offering unprecedented opportunities for efficiency, precision, and innovation. However, many manufacturers face significant challenges, including a lack of data, talent, and leadership. By addressing these barriers and strategically implementing AI, manufacturers can transform their operations, enhance productivity, and stay competitive in the global market.
The Promise of AI in Manufacturing
Artificial Intelligence (AI) holds transformative potential for the manufacturing industry, promising unprecedented advancements in efficiency, precision, and innovation. By harnessing the power of AI, manufacturers can predict equipment failures before they occur, ensure product quality through advanced visual inspections, and optimize supply chain operations for maximum efficiency. The integration of AI not only reduces costs and increases productivity but also enables manufacturers to adapt swiftly to market demands and drive continuous improvement. Embracing AI is essential for manufacturers aiming to maintain a competitive edge and achieve long-term success in an increasingly dynamic and complex global market.
- Operational Excellence AI enhances operational excellence by automating routine tasks, optimizing production schedules, and predicting equipment failures before they occur. Predictive maintenance systems use machine learning to analyze sensor data and anticipate when machinery will need repairs, reducing downtime and maintenance costs. AI-driven process automation and quality control systems ensure consistent production standards and minimize human error, leading to higher productivity and efficiency.
- Value Chain Excellence AI revolutionizes value chain management by optimizing supply chain operations and logistics. AI algorithms analyze vast amounts of data to predict demand accurately, optimize inventory levels, and streamline the flow of materials. This ensures timely delivery of components, reduces lead times, and minimizes inventory costs. AI also enhances supplier management by identifying the most reliable suppliers and anticipating potential disruptions, enabling manufacturers to maintain a resilient and agile supply chain.
- Product & Service Excellence AI empowers manufacturers to innovate and excel in product and service offerings. Advanced analytics and AI-driven insights enable the development of new products tailored to market demands and customer preferences. AI can also enhance product design and testing processes, ensuring higher quality and performance. In the service domain, AI-driven maintenance and support solutions provide proactive service recommendations and remote diagnostics, improving customer satisfaction and loyalty.
- Customer Excellence Despite being primarily B2B, manufacturers can achieve customer excellence through flexibility, adaptability, and personalized production. AI enables manufacturers to implement “segment of one” strategies, producing custom orders tailored to individual customer specifications. AI-driven demand forecasting and production planning ensure that manufacturers can respond swiftly to market changes and customer needs. This flexibility and responsiveness build strong customer relationships and enhance competitive positioning.
- Corporate Excellence AI supports corporate excellence by providing detailed KPIs and guiding OKRs that help monitor, steer, and manage all aspects of the business in real-time. AI-driven analytics offer insights into performance, sustainability, risk management, and financial health, enabling informed decision-making. Leadership teams can leverage AI to identify opportunities for improvement, optimize resource allocation, and drive strategic initiatives. AI also supports sustainability efforts by optimizing energy usage, reducing waste, and ensuring compliance with environmental regulations.
By integrating AI across these dimensions, manufacturers can achieve comprehensive excellence, driving innovation, efficiency, and competitive advantage. Embracing AI is essential for manufacturers aiming to lead in a dynamic and complex global market, ensuring long-term success and resilience.
Addressing Data, Talent, and Leadership Challenges
Addressing the challenges of data, talent, and leadership is crucial for the successful implementation of AI in manufacturing. These barriers, if not adequately managed, can significantly hinder the ability to leverage AI’s transformative potential and drive sustainable growth.
One of the primary challenges is the fragmented and siloed nature of data within manufacturing organizations. Many manufacturers struggle to obtain a comprehensive view of their operations due to inconsistent and incomplete data. To overcome this, companies must invest in modern data infrastructure, including sensors, IoT devices, and advanced data management platforms. These technologies facilitate the seamless collection, integration, and analysis of data from various sources across the production process. Additionally, implementing robust data governance practices ensures data quality, security, and accessibility, which are essential for effective AI utilization. A solid data foundation allows manufacturers to make more accurate and timely decisions, optimizing processes, improving product quality, and enhancing supply chain efficiency.
The second critical challenge is the significant shortage of skilled professionals with expertise in AI, data science, and machine learning within the manufacturing industry. This talent gap makes it difficult to develop and implement AI solutions effectively. To address this, manufacturers need to prioritize talent acquisition and development. This can be achieved by investing in comprehensive training programs, forming partnerships with educational institutions, and creating attractive career paths for AI professionals. Upskilling existing employees through continuous learning opportunities is also crucial to ensure that the workforce remains agile and capable of adapting to new technologies. Collaborations with universities and research centers can further drive innovation and provide access to cutting-edge knowledge and expertise. Building a skilled and knowledgeable workforce empowers manufacturers to design, deploy, and manage AI solutions effectively, driving innovation and staying ahead of competitors.
Learn More: Top 10 Secrets to Succeeding with AI
Our comprehensive ebook provides actionable insights, real-world case studies, and proven strategies to help you integrate AI into your operations and drive growth.
Download Now
Leadership and strategic vision play a pivotal role in the successful implementation of AI. Many manufacturing leaders may lack the understanding or commitment to drive AI initiatives, resulting in fragmented efforts and missed opportunities. Leadership teams must champion AI transformation by articulating a clear and compelling vision that aligns with business goals and market demands. Fostering a culture of innovation and collaboration is essential, as it encourages cross-functional teams to work together on AI projects. Investing in leadership development programs that focus on digital transformation and change management equips leaders with the skills needed to navigate the AI journey successfully. Visionary leadership provides the direction and motivation necessary to drive AI initiatives forward, ensuring that AI investments deliver measurable value and support long-term growth.
Furthermore, manufacturers should consider building an ecosystem of partners, including technology providers, academic institutions, and industry consortia. Implementing AI in isolation can be daunting and may lead to suboptimal outcomes. Developing partnerships enables knowledge sharing, access to advanced tools and platforms, and collaborative innovation. This ecosystem approach accelerates AI implementation and enhances the overall effectiveness of AI initiatives. By leveraging the expertise and resources of partners, manufacturers can overcome internal limitations and drive more significant innovation and value creation.
By addressing these challenges related to data, talent, and leadership, manufacturers can unlock the full potential of AI, driving transformation and ensuring competitiveness in a rapidly evolving industry. Investing in data infrastructure, developing a skilled workforce, and fostering visionary leadership are critical steps toward realizing the promise of AI in manufacturing.
Key Use Cases for AI in Manufacturing
Harnessing the power of AI across the five areas of excellence—Operational Excellence, Value Chain Excellence, Product & Service Excellence, Customer Excellence, and Corporate Excellence—can drive significant value for manufacturers. Here are the top AI use cases in manufacturing, with additional bonus use cases for comprehensive coverage.
- Predictive Maintenance (Operational Excellence)
AI can predict equipment failures before they occur by analyzing sensor data and identifying patterns that indicate potential issues. This reduces unplanned downtime, extends the lifespan of machinery, and lowers maintenance costs, ensuring smooth and efficient operations. - Quality Control and Inspection (Operational Excellence)
AI-driven visual inspection systems use image recognition to detect defects and ensure product quality. These systems are more accurate and consistent than human inspectors, reducing waste, improving product reliability, and enhancing customer satisfaction. - Inventory Management (Value Chain Excellence)
AI optimizes inventory levels by analyzing sales data, market trends, and supply chain information. This minimizes stockouts and overstock situations, reduces carrying costs, and improves overall supply chain efficiency. - Demand Forecasting (Value Chain Excellence)
AI algorithms predict customer demand with high accuracy by analyzing historical sales data, market conditions, and external factors. This enables better planning, resource allocation, and reduces excess inventory, aligning production with market demand. - Supply Chain Optimization (Value Chain Excellence)
AI enhances supply chain management by identifying the most efficient routes for material transportation and predicting potential disruptions. This leads to reduced lead times, lower transportation costs, and increased supply chain resilience. - Product Design and Development (Product & Service Excellence)
AI accelerates product development by analyzing customer feedback, market trends, and performance data. This allows manufacturers to create innovative products that meet customer needs and maintain a competitive edge in the market. - Predictive Quality Analytics (Product & Service Excellence)
AI analyzes production data to identify factors that affect product quality. By addressing these factors proactively, manufacturers can improve product consistency, reduce defects, and enhance customer satisfaction. - Personalized Production (Customer Excellence)
AI enables manufacturers to implement “segment of one” strategies, producing custom orders tailored to individual customer specifications. This flexibility and adaptability meet the growing demand for personalized products, enhancing customer loyalty and differentiation. - Adaptive Manufacturing (Customer Excellence)
AI-driven adaptive manufacturing systems adjust production processes in real-time based on customer orders and market demand. This ensures optimal resource utilization and rapid response to changing customer needs, improving overall efficiency and satisfaction. - Sustainability Management (Corporate Excellence)
AI optimizes energy usage, reduces waste, and ensures compliance with environmental regulations. This supports sustainability initiatives, reduces operational costs, and enhances the company’s reputation for environmental responsibility. - Risk Management and Compliance (Corporate Excellence)
AI helps in monitoring regulatory changes, automating compliance processes, and identifying potential risks. This ensures adherence to regulations, reduces the risk of non-compliance, and enhances overall corporate governance. - Performance Monitoring and Optimization (Corporate Excellence)
AI provides detailed KPIs and real-time analytics to monitor and optimize manufacturing performance. This allows leadership to make data-driven decisions, improve operational efficiency, and drive continuous improvement.Bonus Use Cases:
- Workforce Management (Operational Excellence)
AI optimizes workforce scheduling and task allocation by analyzing employee performance and production needs. This improves labor productivity and reduces operational bottlenecks. - Digital Twin Technology (Operational Excellence)
AI-powered digital twins create virtual replicas of physical assets to simulate and optimize manufacturing processes. This allows for real-time monitoring, predictive maintenance, and scenario testing, enhancing overall efficiency and innovation. - Augmented Reality (AR) for Training (Corporate Excellence)
AI and AR technologies provide immersive training experiences for employees, improving skill development and safety. This helps in quickly upskilling the workforce and maintaining high standards of operation. - Customer Sentiment Analysis (Customer Excellence)
AI analyzes customer feedback from various channels to gauge sentiment and identify areas for improvement. This helps manufacturers tailor their products and services to better meet customer expectations. - Autonomous Robotics (Operational Excellence)
AI-driven robots automate repetitive tasks, enhancing precision and productivity on the factory floor. This reduces labor costs and increases operational efficiency. - Market Trend Analysis (Product & Service Excellence)
AI analyzes market data to identify emerging trends and consumer preferences. This helps manufacturers stay ahead of the competition by aligning their product development strategies with market demands.
By integrating AI across these key use cases, manufacturers can achieve comprehensive excellence, driving innovation, efficiency, and competitive advantage. Embracing AI is essential for manufacturers aiming to lead in a dynamic and complex global market, ensuring long-term success and resilience.
Influential Success Stories in AI-Driven Manufacturing
The AI revolution in the manufacturing industry is already underway, with leading companies demonstrating the transformative power of artificial intelligence. These success stories illustrate the significant benefits of AI adoption, from enhanced operational efficiency to groundbreaking innovations. As these examples show, embracing AI is crucial for manufacturers who wish to thrive in a competitive and dynamic market.
Siemens – Germany (Predictive Maintenance)
Challenge: Siemens, a global leader in industrial manufacturing, faced challenges in maintaining its extensive range of machinery and equipment. Unplanned downtime and maintenance costs were significant issues that impacted productivity and profitability.
Solution: Siemens implemented an AI-driven predictive maintenance system that uses machine learning algorithms to analyze sensor data from equipment. The system predicts potential failures before they occur, allowing for proactive maintenance scheduling. This approach helps identify patterns and anomalies in equipment behavior, reducing the likelihood of unexpected breakdowns.
Benefits: The AI-driven predictive maintenance system has significantly reduced unplanned downtime and maintenance costs for Siemens. The company has seen improved equipment reliability and increased productivity. By adopting this proactive maintenance strategy, Siemens has enhanced its operational efficiency and strengthened its position as a leader in industrial manufacturing.
Foxconn – China (Quality Control)
Challenge: Foxconn, one of the world’s largest electronics manufacturers, needed to ensure high-quality standards for its products. Manual inspection processes were time-consuming and prone to human error, leading to potential defects and production delays.
Solution: Foxconn deployed AI-driven visual inspection systems to automate the quality control process. These systems use advanced image recognition algorithms to detect defects in real-time during the production process. By analyzing images of products, the AI system can identify even the smallest defects with high accuracy.
Benefits: The implementation of AI-driven quality control systems has significantly improved product quality and reduced inspection times at Foxconn. The automated systems have increased the accuracy and consistency of inspections, minimizing the risk of defective products reaching customers. This has enhanced Foxconn’s reputation for quality and reliability in the electronics manufacturing industry.
FANUC – Japan (Supply Chain Optimization)
Challenge: FANUC, a leading manufacturer of robotics and industrial automation equipment, faced challenges in optimizing its complex supply chain. Coordinating the flow of materials and components across multiple suppliers and production facilities was a significant logistical challenge.
Solution: FANUC implemented an AI-powered supply chain optimization system that uses machine learning algorithms to analyze vast amounts of data from across the supply chain. The system predicts demand, optimizes inventory levels, and identifies the most efficient routes for material transportation. By leveraging AI, FANUC can respond to changes in demand and supply conditions in real-time.
Benefits: The AI-driven supply chain optimization system has enhanced FANUC’s operational efficiency and reduced costs. The company has achieved better inventory management, reduced lead times, and improved overall supply chain resilience. This has enabled FANUC to meet customer demands more effectively and maintain a competitive edge in the global market.
Key Recommendations for Successful AI Transformation in Manufacturing
To successfully implement AI in manufacturing and unlock its transformative potential, it is crucial to address several strategic areas. Here are key recommendations to guide manufacturers on their AI transformation journey:
Invest in Data Infrastructure
- Build a Robust Data Foundation: Invest in modern data infrastructure, including sensors, IoT devices, and data management platforms, to enable seamless data collection, integration, and analysis. Ensure data quality, security, and accessibility through robust data governance practices.
- Utilize Advanced Analytics: Leverage AI-driven analytics to gain insights from collected data, driving informed decision-making and continuous improvement.
Develop AI Expertise
- Talent Acquisition and Development: Prioritize the acquisition and development of skilled professionals with expertise in AI, data science, and machine learning. Offer comprehensive training programs and create attractive career paths to build a knowledgeable workforce.
- Continuous Learning and Innovation: Foster a culture of continuous learning and innovation by providing employees with opportunities for upskilling and collaborating with universities and research centers.
Cultivate Visionary Leadership
- Champion AI Transformation: Leadership teams must articulate a clear and compelling vision for AI adoption that aligns with business goals and market demands. Promote a culture of innovation and collaboration across all levels of the organization.
- Leadership Development Programs: Invest in leadership development programs focused on digital transformation and change management to equip leaders with the necessary skills to drive AI initiatives.
Leverage Ecosystem Partnerships
- Build a Network of Partners: Develop an ecosystem of partners, including technology providers, academic institutions, and industry consortia, to bridge capability gaps and accelerate AI adoption. Collaborate with partners for knowledge sharing, access to advanced tools, and collaborative innovation.
- Joint Ventures and Collaborations: Engage in joint ventures and collaborations to co-develop AI solutions and leverage external expertise for complex projects.
Align AI Initiatives with Business Goals
- Strategic Use Case Identification: Identify and prioritize AI projects that align with strategic business objectives. Focus on high-impact use cases that deliver measurable business value and build momentum for broader AI adoption.
- Integration Across Business Units: Ensure that AI initiatives are integrated across all business units and functions, fostering a holistic approach to AI transformation.
Implement Continuous Measurement and Improvement
- AI Maturity Index: Utilize tools like Digitopia’s AI Maturity Index to continuously assess and benchmark AI capabilities. Regularly review progress, adjust strategies, and ensure that AI initiatives are delivering the expected value.
- Data-Driven Decision Making: Monitor performance through detailed KPIs and real-time analytics, enabling data-driven decision-making and continuous optimization of AI initiatives.
Promote Sustainability and Corporate Responsibility
- Sustainable AI Practices: Implement AI solutions that optimize energy usage, reduce waste, and ensure compliance with environmental regulations. This supports sustainability efforts and enhances the company’s reputation for environmental responsibility.
- Ethical AI Adoption: Ensure that AI technologies are adopted ethically, with considerations for privacy, security, and fairness. Establish guidelines and frameworks for responsible AI use.
By addressing these strategic areas, manufacturers can effectively navigate the challenges of data, talent, and leadership, ensuring successful AI transformation. Embracing AI across operational, value chain, product, customer, and corporate excellence will drive innovation, efficiency, and competitive advantage, positioning manufacturers for long-term success in a dynamic global market.